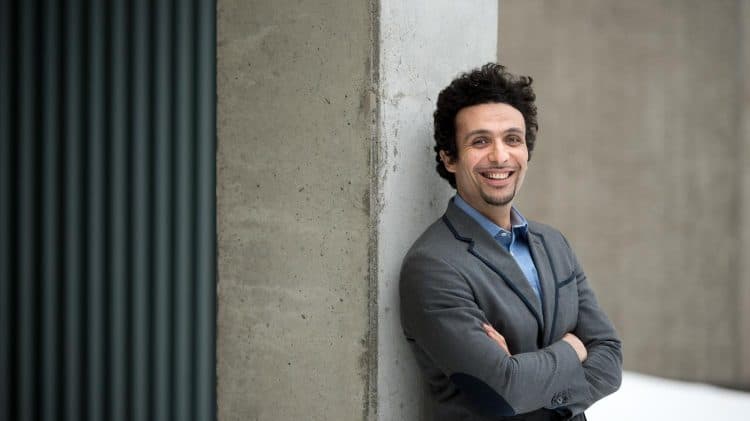
Beyond communications engineering in 6G
Enthusiastic and expressive, Mehdi Bennis has a way of pulling a listener into his field of excitement without resistance. The Centre for Wireless Communication associate professor has been wrapping his head around the 6G vision and while thinks the real revolution is yet to come, he has a pretty good idea where it will come from.
“Google’s federated learning is a low-hanging fruit”, says Bennis, but there is more to come with federated knowledge distillation.
While 6G is still years in the future, we can count on a few things as certainties, when it does come. First, the amount of data generated, transmitted and analyzed is going to grow exponentially. Second, we will have super-high transmission rates to whisk that data around in the ether. Then the two big buzzwords of the moment—artificial intelligence and machine learning—are usually thrown in the mix as all-purpose solutions. But how do we get to the promise of the future, to a digital mirror world where we can simulate our complex systems before building them for real, to having the ability to reason and plan instead of mere pattern matching and curve fitting as done today.
Machine learning and its marriage with communication are what keep Bennis busy at the University of Oulu. Coming from a background in communications engineering, Bennis earned his PhD in Oulu in December 2009 and stayed as a post-doc researcher at CWC. He now runs ICON, a research group of 12 talented researchers whose focus is on the intersection of machine learning, communication and control, focusing on how to enable intelligent communication based on limited data and extracted knowledge. Bennis says that he is incorporating many disciplines in his work, drawing from such fields as game theory, control theory, financial theory and neuroscience, among others.
“During my visit in Princeton in 2017, I began looking at tail distributions,” Bennis says, gesturing with his hands, creating a Bell curve in the air, and then focusing on a tiny sliver of its end. “The things that happen very rarely, but when they do, a lot of stuff goes wrong.”
“So, it’s things like earthquakes and hurricanes and the like, rare, or hard-to-predict events that cause massive repercussions. I was not convinced by the focus back then on running time-consuming simulations without gaining any basic understanding of URLLC. So I decided to bring in the concept of tail distribution thinking into wireless, especially as it relates to the reliability of communication networks. And so my focus became how to bring in this awareness of tail distribution to wireless, machine learning, and, basically, how to manage risk. Now the entire industry talks about tails,” Bennis adds.
Brute force is only useful to an extent
Bennis felt that the conversation in wireless was—and still is—revolving around data and data transfer speeds. Machine learning needs vast amounts of data to create models of behaviour. Problems arise when you don’t have enough data for it, or the amount of data is so huge that networks can’t keep up with it in a meaningful, low-latency kind of way.
“It’s this idea of training a model with tons of data but this is very inefficient and bandwidth-consuming, not to mention horrible in terms of privacy concerns. What’s actually smarter is to have all the devices, such as your mobile phone, transmit a model instead of data. Raw data stays on your device, and only the model is updated. The beauty is that devices collaboratively train their model in a privacy-preserving manner. And this has significant implications and pervades to other areas such as medicine, epidemics, and so on,” Bennis explains.
And this is what Google has termed ‘federated learning’: devices update the model using local data and upload this locally trained model back to the central server, where all the updates from all the devices are aggregated.
“ICON is the first group to investigate federated learning in the context of wireless communication in the context of 6G, an area that is rapidly evolving. But we need much more,” Bennis says.
Because even in this approach we are still trying to do pattern matching and correlation. As Bennis sees it, the important thing is to extrapolate, not interpolate. This is why we need to look into neuroscience, physics, and other areas.
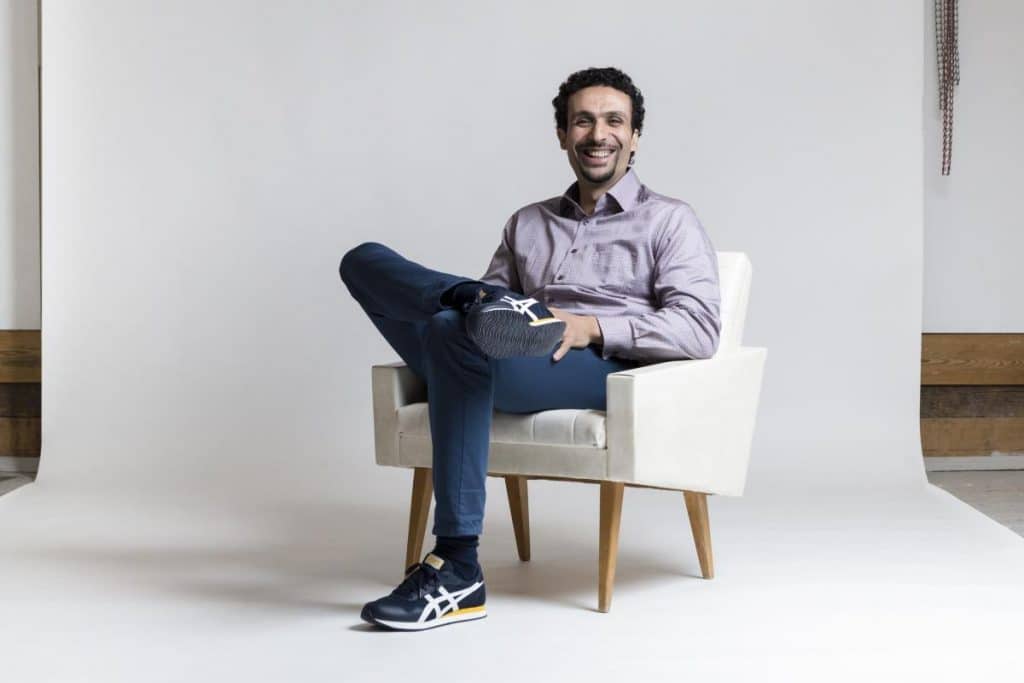
“Among these core concepts causality is key to meaningful and interpretable artificial intelligence and machine learning. We need to understand and teach the algorithms what causes the actual phenomena? This is how we get to building mirror worlds, digital twins of actual things, simulating a complex factory line with dozens of robots before actually deploying it in the real world,” Bennis enthuses.
“The answer is not more bandwidth and taking an engineering centric only approach as is currently proposed. Machine learning solutions are still brute-forcing it. Quoting [VR expert and roboticist] Steven LaValle, who speaks about engineering a perceptional illusion. In a VR situation, you need to trick the human brain to do the work for you. In other words, you don’t need the maximum amount of data at the maximum speed, you only need enough of it. You need the system to distill out the irrelevant and unnecessary stuff and focus on the essential things,” Bennis says.
From 6G vision to Vision X
And this distillation of things is what drives Bennis now in his new vision for the future (“I call it ‘Vision X’”) which he says will be the revolution in 6G.
“You don’t transmit the raw data, you only transmit the model. But model parameters can also be heavy. Now, model compression or distillation will make them smaller. It’s trying to use the absolute minimum resources, like bandwidth and data, to achieve maximum results reliably,” Bennis explains. And this is the paradigm change.
Bennis gives a teacher-student interaction as an analogy. The teacher is passing on knowledge to the student and supervising them so they will reach the same result as the teacher. Teachers need to distill their knowledge for this to happen. In the analogy, the teacher can be a base station and the student can be a device, a cell phone or a robot. Then, these clusters of devices can train their models collectively, distill out the useless information and only proceed with the necessary information to carry out a task or achieve a goal.
“And this is how we have to design our future systems. There is structure in the world and we need to understand it. AI is here and it is rapidly evolving. So, let’s connect it with neuroscience and talk about attention and causality. Let’s connect it with physics and talk about symmetry. Federated learning is great but it is really the low-hanging fruit of 6G. Federated distillation is one step towards the coming revolution, but there is a whole lot yet to come. Stay tuned,” Bennis says.