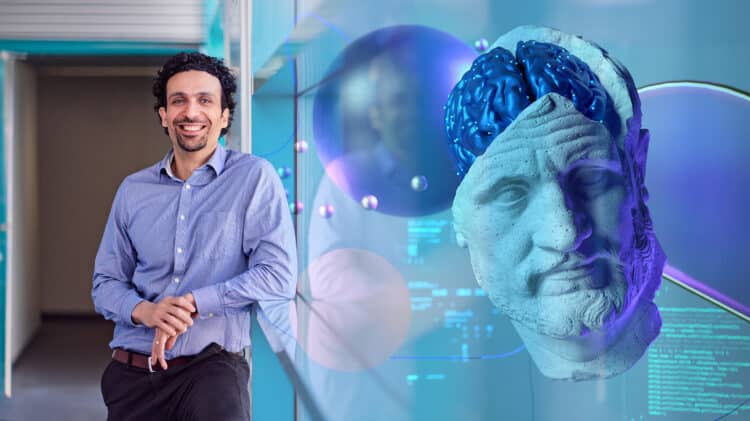
What should a robot say to another robot? Future networks must imagine and plan, says Mehdi Bennis
Professor Mehdi Bennis has been thinking a lot about a word that was seemingly everywhere at the height of the Covid-19 pandemic: resilience. Resilience is the ability to protect oneself from harm but also, and maybe more importantly in Bennis’ sense of the word, the ability to bounce back and transform after getting harmed or suffering from stressors. Bennis started looking at networks, wireless communication, machine learning, AI and virtually everything in his field through the perspective of resilience.
“Reliability is a big word we associate with networks, as is robustness. Resilience must be something else. Otherwise, we would be satisfied with robustness and reliability, right? Designing a network based on resilience gives you a totally different way of thinking about and designing networks, with a totally different set of requirements,” Bennis says.
Resilience is a concept commonly used in cyber security in terms of protecting a network from cyber attacks, injections of malicious code and so on, but Bennis’ view of the term is far more encompassing. To explain, he goes back to the concept he has been working on for many years during his time in 6G Flagship, “Vision X.”
When 6G research began in earnest, the thing that was taken for granted was that in the future, we would have faster networks, more base stations, higher frequencies, ultra-low latency, incredible computing power in the cloud and on the edge to process fantastic amounts of data. Bennis says he wanted to look at things from a totally different angle. Instead of counting on the predicted ability to process tons of data incredibly fast to create, say, self-driving cars, Bennis looked at the human brain for inspiration, which has the ability to infer things from very few pieces of information.
“Vision X is a blueprint for semantic communication. When we humans communicate, we don’t bombard each other with every bit of information we have. Instead, we communicate the essence, the gist of things, which the receiver is able to interpret. Vision X was built on the idea that smaller and smarter equals better,” Bennis says.
Bennis brings up large language models, or LLMs, as a case in point.
“LLMs opened the eyes of the world to see that we can use them for many tasks. But so far, they rely on statistics. They lack the power to think, to imagine, to ask ‘what if’ questions, which we humans do. And yes, you can think of humans as being LLMs in their own right, but LLMs that think instead of replicate.”
‘What if’ questions are very important in terms of resilience. What if things go wrong? What if that rock I see in a dark forest is actually a bear? As a human, I can make plans for a getaway in such a situation. I can also move in the world, physically find another angle to look and see that it is indeed a large rock and not a bear. What about networks, or robots, or sensors?
“If I have partial information, I have to communicate with other people or other sources of information. Robots and LLMs need to do this as well. Imagine you have a large house and a fleet of cleaning robots. It doesn’t make sense for all the robots to flock in the same room, so they have to communicate who is going where to clean. They have to move in the world, perceive and create plans or schemas. Right now, AI is pure recognition or perception. What is lacking is what humans do: sense, perceive, abstract, imagine, and plan. This cycle is the essence of Vision X,” Bennis says.
Quantifying resilience
Resilience has a built-in assumption that something will go wrong, and the question becomes how and how fast do you recover from it.
“There are two schools of thought about resilience, basically. One is that you get knocked down, and you get back up, back to the same state you were in before the event. The other is that you get knocked down, and you come back evolved, changed by the event. For networks, the idea of evolution becomes very important,” Bennis ponders.
Bennis takes another example from the human brain. Resilience in the brain is often called plasticity, the ability of the brain to rewire itself to take over functions of another part of the brain that may have been damaged. The question becomes how to build this type of resilience in a network.
“We need to be able to quantify resiliency because if you can’t quantify it, you can’t really even talk about it. Some of the key metrics are recovery time and sustainability or durability. These metrics have to be used when stress testing a network, and this is what I am doing with my research group, developing the mathematics of resilience,” Bennis says.
Bennis and his group, ICON, are doing many proof-of-concept pilots as well as publishing papers. Currently, Bennis and his group are working on a paper to position Oulu as the leader in the resiliency concept. The task is not simple: as with many other things, resilience comes with a cost. There are requirements for mitigating delay, having high computing power available and so on. In essence, you are trying to create a way to think very fast.
“And to think you need also to know what you don’t know, what information is missing. This requires a robot or LLM to understand another robot or LLM. And so we have to find what is the most important prompt a robot or LLM gives to another robot or LLM,” Bennis muses.